The first part of this article, dedicated to the three pillars of maturity for the data-driven organization, focused on the organization. In this second part, we consider architecture and governance.
Architecture: Focus on self-service, flexibility, and usage diversity
Because of the extreme diversity of use cases, and although there are exceptions that prove the rule such as financial planning or customer data platforms, the data landscape has not been packaged into pre-configured applications. Even though major technology waves have sparked advances in the data world (from data warehousing to business intelligence, and then to big data, the cloud and data science), it is the responsibility of the data teams within their organization to ride these waves (or not) towards trajectories that not only fit the context of their organization and its various use cases but also allow it to move forward as technology, organizational and functional needs evolve. Mastering architecture is therefore mandatory.
To achieve this, the Cloud has been established as a core enabler, because it fosters flexibility and agility, but also democratizes access to technologies, their experimentation and scaling, even for small organisations.
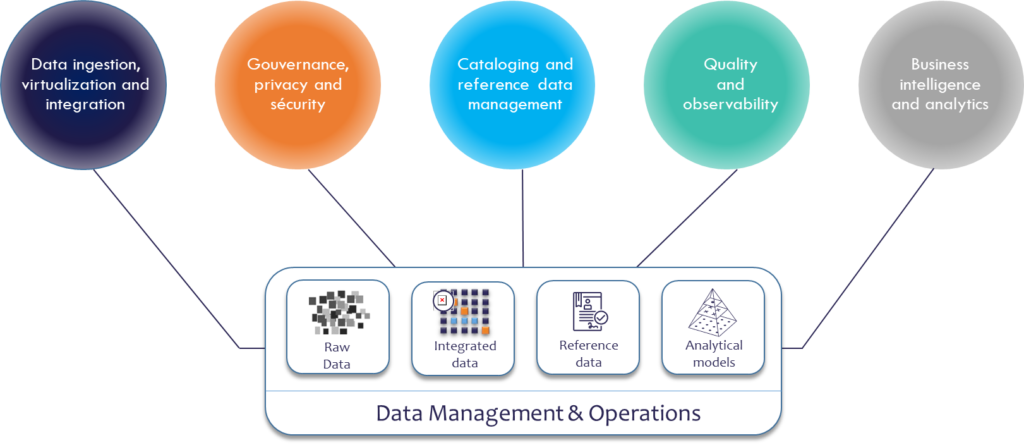
Historically organized around a central database that enables self-service access to a wide range of users, the lower layer of the architecture had to evolve into a modularized environment that can address data variety, from raw data to reference data or data that has been modelled specifically for certain use cases. The platform is also being virtualized, so that a centralized copy of data is not the only option, also allowing data decentralization or real-time data processing.
Upstream, the architecture should enable data sourcing (including but not only ingestion) and integration, which remains a back-office task managed by DataOps. Downstream, it should enable self-service access to the masses, more sophisticated analysis for data science, and programmatic access to supply data and trigger actions in business applications.
On top of those three pillars, comes the fourth dimension for governance, reliability, and data accessibility. It aims to take control over data, but also to bring more transparency and documentation to data for self-service use and operationalization. Capabilities include access and security management, provisioning, data protection, cataloging capabilities, together with data quality and observability, the potential Achilles’ heel of data initiatives whose data lakes are at risk to turn into swamps.
Such transparency requires building a semantic layer that allows to reference the data, understand their relationship and origin, and connect to their business definition and context. The development of this dimension has been hindered until now because it required significant human efforts, with a declarative approach. Recent technological innovations allow for automation and computational intelligence, potentially propelling this dimension at the center of a modern data platform.
Setting the framework for governance and quality
There is general agreement that data governance is a cornerstone of a successful data initiative. But unfortunately, it is frequently the weakest link, as it does not stop with a framework, you then need to convey and enforce its principles. A data governance program is cross-functional by nature and must establish roles and responsibilities, policies, standards, and processes, along with rules and controls. It is a transformation that requires change management, recognizing the need and consequences, and engaging stakeholders in its realization.
The diagram below presents a framework for governance, strongly but loosely based on Dave Wells’ modern governance framework.
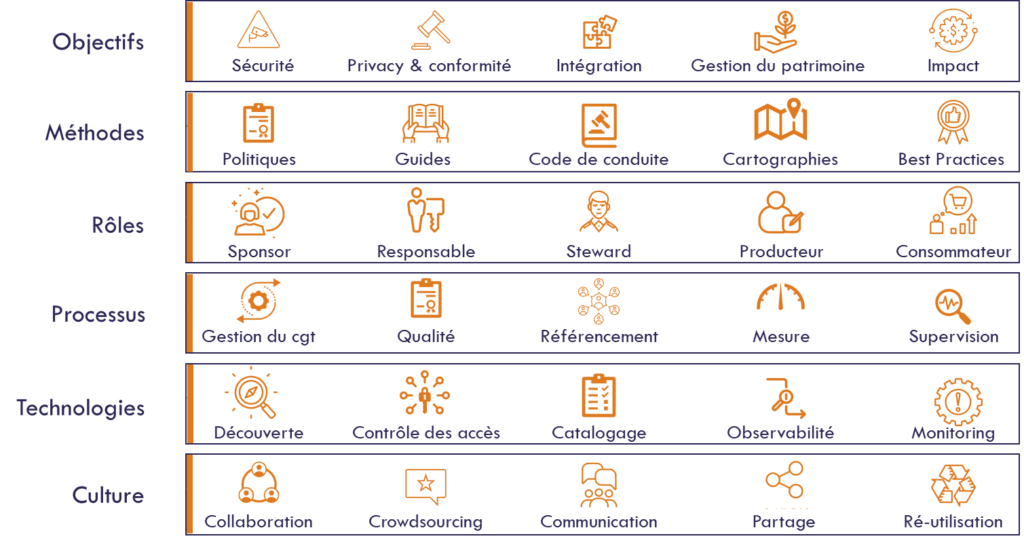
A governance program should start with the why and how to ensure alignment with the organisation’s challenges and vision. This is a prerequisite for obtaining the sponsorship of top management and the commitment of all stakeholders.
Next, the guiding principles and the related methods for formalizing them must be designed, such as codes of conduct or the ways in which the data will be inventoried and mapped. Since governance is about establishing a system of decision-making entities rather than a single decision-making center, roles and accountabilities need to be clearly defined and assigned, and how they fit into the organization. As initiatives grow in size and impact, the roles become more numerous and diverse.
Execution can then take place using the defined framework and roles. This entails defining processes and controls, such as establishing privacy by design, ensuring and measuring data quality, and systematically referencing data.
Even if they are only a means to an end, technologies play an increasing role in scaling up. Data is overwhelming and constantly changing, while resources dedicated to governance are limited. Technologies must allow linking dynamically the rules to the actual data, and to the data owners and stewards who are in control.
Finally, data is a team sport, while silos destroy its value. Data governance must therefore provide and foster a behavioral framework that stimulates appropriation, collaboration, sharing and adherence to ethical principles. This unifying role, aimed at establishing participative governance across stakeholders, is the greatest challenge for data governance, but also more generally for the development of the data economy. The implementation of GDPR for data privacy in Europe gave us an interesting overview of the issues, challenges, and impacts.
See also the first part of this article focused on the organisation.
This article is based on a presentation made for the keynote of the KPC consulting data days in Paris. Click below to access the full presentation.